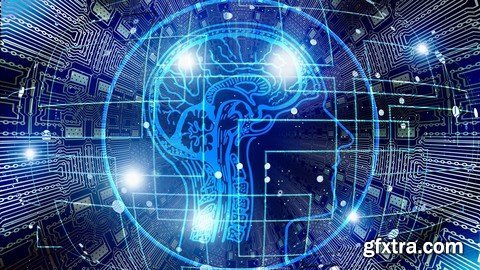
Learn to Implement Classification Models in Scikit-learn ( sklearn ) : A Python Artificial Intelligence Library
Artificial intelligence and machine learning are touching our everyday lives in more-and-more ways. There’s an endless supply of industries and applications that machine learning can make more efficient and intelligent. Supervised machine learning is the underlying method behind a large part of this. Supervised learning involves using some algorithm to analyze and learn from past observations, enabling you to predict future events. This course introduces you to one of the prominent modelling families of supervised Machine Learning called Classification. This course provides the learners with the foundational knowledge to use classification models to create business insights. You will become familiar with the most successful and widely used classification techniques, such as Support Vector Machines. Naive Bayes Decision Tree Random Forest K-Nearest Neighbors Neural Networks Logistic Regression You will learn to train predictive models to classify categorical outcomes and use performance metrics to evaluate different models. The complete course is built on several examples where you will learn to code with real datasets. By the end of this course, you will be able to build machine learning models to make predictions using your data. Get ready to do more learning than your machine! Happy Learning.
TO MAC USERS: If RAR password doesn't work, use this archive program:
RAR Expander 0.8.5 Beta 4 and extract password protected files without error.
TO WIN USERS: If RAR password doesn't work, use this archive program:
Latest Winrar and extract password protected files without error.